First, there was the Human Genome Project. The turn-of-the-century, international research program led to the sequencing and mapping of humanity’s entire genetic makeup at a fundamental level, paving the way for thousands of discoveries and treatments to address the world’s trickiest diseases.
Now, chemical and biological “maps” have advanced far beyond what the Human Genome Project achieved, digging deeper into the inner workings of physics and the human body. And new technology like machine learning and AI has sparked a revolution in how complex and voluminous these roadmaps can be.
Last week, the Nobel Prize in chemistry went to three scientists who developed and used the neural network AI program AlphaFold to predict the intricate folding patterns of proteins and create proteins of their own. The researchers — Demis Hassabis and John Jumper of Google’s DeepMind in the U.K., and the University of Washington’s David Baker — have touted the program’s potential for unprecedented drug discovery and development.
“A tech company winning the Nobel Prize in chemistry is the dawn of a new era. And while they map the chemical structure of proteins, what we are trying to do is map immune systems in living organisms — so it’s more complex.”

Noam Solomon
CEO, Immunai
AlphaFold is far from the only use of machine learning to unveil the mysteries of biology. The life sciences industry is awash with companies leveraging big data and predictive analytics to decipher critical pathways and catalog them as an advanced tool for drugmakers. With the range of capabilities the mapping tools offer — from revealing the complexities of the immune system and how drugs interact — these technologies could change the way drug development is done.
Mapping the immune system
Immunology is a hot target in biotech and pharma, and with the success of megablockbusters like Humira as a guiding light, venture capital investments in autoimmune conditions reached $1.7 billion in the first half of 2024 alone.
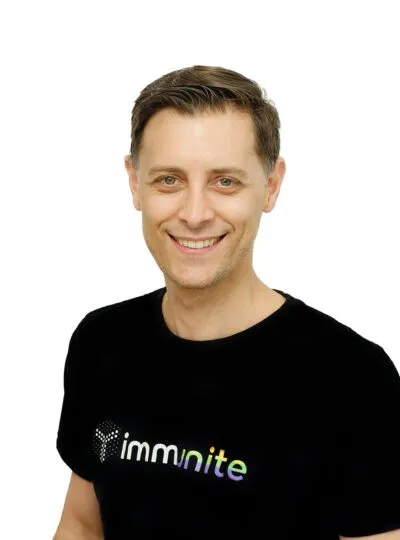
But when it comes to the complexities of the immune system and what causes it to function improperly, the data is so complicated that vast swaths of it are still misunderstood. Developing new treatments that improve upon their predecessors becomes more challenging and expensive over time, said Noam Solomon, CEO of Immunai, which boasts the largest knowledge base of cell-level immunology in the world.
The goal of Immunai’s AI platform, called Amica, is to create a map of the immune system at the single-cell level and demonstrate how those on and off switches respond to different drugs, Solomon said. Because of the breadth of the immune system’s involvement with human disease, the implications could span from oncology to neuroscience and many other areas. And Immunai wants to make the drug development process more directed toward the end outcome.
“The reason you have such poor response rates and approval rates for therapeutics is because different people’s immune systems behave differently, and the way biotech and pharma companies are developing the drugs is from very simplistic and reductionist preclinical data,” Solomon said. “There are a lot of decisions for a drug, from mechanism of action to indications to patient selection and combinations — we leverage big data engineering and an AI model to answer and explain these questions.”
What that map provides is not just a view of all the connections within the immune system but also a path through the drug development process — like a robotic assistant who can point researchers and developers in the right direction.
Immunai recently expanded a partnership with pharma giant AstraZeneca to guide clinical decision-making using the immune system map. The companies have worked together since 2022 to bring compounds through development, and the two will focus the collaboration on oncology and immunology.
If Immunai’s platform is the GPS to navigate the immune system, Solomon said, then a drugmaker like AstraZeneca provides the car — the clinical trial infrastructure. Still, it can take a couple years to build the trust of a more traditional pharma company and demonstrate that a new platform is viable.
And drugmakers need to understand the limitations of technology as much as the possibilities, Solomon said.
“We need to view AI as an augmentation of capabilities,” he said. “Precision medicine right now is essentially built on the premise that there are a lot of different types of data, very high-dimensional, that the human brain can’t cope with or make sense of, but computers are exactly geared toward that.”
Down the road, maps like Immunai’s will just be how the job is done, Solomon said. DeepMind’s Nobel recognition is a testament to that.
“A tech company winning the Nobel Prize in chemistry is the dawn of a new era,” Solomon said. “And while they map the chemical structure of proteins, what we are trying to do is map immune systems in living organisms — so it’s more complex.”
Applying physics
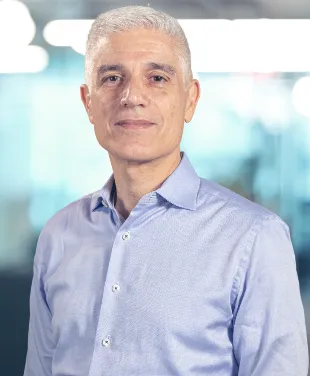
Ramy Farid, CEO of the physics-based software company Schrödinger, views the DeepMind Nobel Prize as a step in the right direction for transformative drug development advances, but he has a bigger question on his mind: “Now what?”
Like the Human Genome Project, AlphaFold solves a specific problem by exploring the existing structures governing druggable targets. But it’s still just a step in the right direction, Farid said.
“Knowing the sequence of the whole human proteome tells us some information, and it’s a real accomplishment — but you can’t design drug molecules with this technology,” Farid said. “Knowing the structure is important, but we also need technology to understand how molecules bind to proteins.”
Every achievement begets more paths forward, and Schrödinger has for years been building its own drug discovery software that provides a molecular understanding of diseases and their potential treatments. Among other capabilities, their physics-based approach applies mathematical constants to molecular structures to create a “map” of how they will bind to one another, which is an important step in drug discovery and development. The company now has its own pipeline of candidates across oncology, immunology and neurology, as well as partnerships with Bristol Myers Squibb, Gilead Sciences and Eli Lilly.
Leading the charge with the company’s drug development arm is Karen Akinsanya, president of R&D and a former research leader at Merck & Co, where she worked with a program that was faltering until Schrödinger’s software helped pave the way.
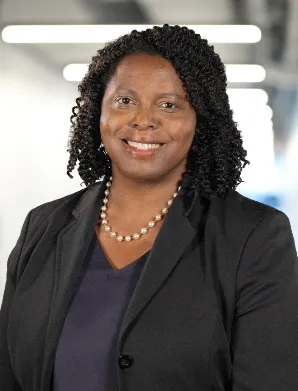
“Essentially, we had hit a brick wall, and Schrödinger showed how powerful the technology was to go beyond the chemical space that the industry had all been working on and expand it,” Akinsanya said. “And as a value growth story, the idea was that with unlimited access to the platform, we should be able to at least attempt to solve some of the biggest challenges in drug discovery.”
But Schrödinger, as well as DeepMind and other companies in the space, needs to understand the limitations of this kind of technology, Farid said.
“AlphaFold has sparked a lot of interest in methods like this,” Farid said, “And while computational structures are great, you’re going to keep needing experimental structures, too, because it’s not perfect.”
Circling back to the Human Genome Project, Akinsanya views current advances like AlphaFold and Schrödinger’s platform as necessary steps in a long journey.
“The Human Genome Project was an incredible moment in history, and a lot of things have come out of that — but it wasn’t the solution to curing every disease we thought it would be,” Akinsanya said. “But so much has happened in the last 25 years that did come from that project, like mRNA and rapid sequencing — these are breakthroughs as a result of that breakthrough, and that’s what we’re all excited about in this space, as well.”