Diagnosis of neurodegenerative conditions like Alzheimer’s disease has progressed significantly in recent years due to various biomarkers. For the most part, however, diagnosis typically depends on documenting mental decline, and at that point, a lot of the damage is already done.
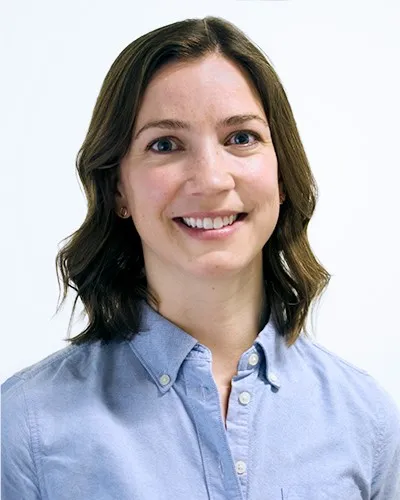
With Alzheimer’s becoming a growing concern globally due to aging populations, the need for ways to detect and monitor cognitive decline is becoming increasingly paramount. And as drug companies continue to search for treatments and cures for Alzheimer’s, they need to find patients for their trials. That isn’t easy.
Machine-learning tools assessing speech could be effective in neurodegenerative disease detection. These speech-based digital biomarkers aren’t invasive and cost a lot less than cerebrospinal fluid taps that have been used to diagnose Alzheimer’s and other forms of dementia.
A 2016 study published in the Journal of Alzheimer’s Disease showed that diagnoses could determine more than 81% accuracy in distinguishing individuals with Alzheimer’s from those without, based on short samples of their language on a picture description task. The study outcome led to the launch of WinterLight Labs, which has developed a tablet-based technology that assesses cognitive health by analyzing hundreds of language markers from short snippets of speech.
“Since starting out, we have built out our data collection app, not just in terms of how we analyze speech, but having a user-friendly interface to collect and analyze that data on our servers,” Jessica Robin, director of clinical research at WinterLight Labs, says. “These classification models are well-suited to pre-screening and diagnostics because they are fast, automated and objective.”
"We take a dual approach where we look both at how a person says something and what they’re actually saying, and that gives us insight into the disease."

Jessica Robin
WinterLight Labs director of clinical research
Clinicians have been using speech patterns in their assessments for many years to determine whether a patient is having trouble communicating, which can help them gain insights into cognition or assess mood, motor and respiratory function.
While predicting whether someone has Alzheimer's is important, it’s only a starting point, Robin says. The next priority is to characterize how these language changes evolve with disease progression, and that’s where WinterLight has been focusing a lot of its efforts.
“That will really give us the targets for clinical research to start seeing if we can slow that decline. Can we even potentially reverse some of these language changes, and could we use speech methods to detect those changes with more sensitivity or earlier in the trial?” Robin adds.
Here, Robin discusses the importance of speech as an indicator of neurodegenerative disease, how WinterLight is measuring the impact of disease on speech patterns, and what these findings could mean for clinical trials.
This interview has been edited for length and clarity.
PharmaVoice: Can you describe how you are applying your technology platform to identify neurodegenerative disease and its progression?
Jessica Robin: We take a dual approach where we look both at how a person says something and what they're actually saying, and that gives us insight into the disease. In Alzheimer's disease, for example, the linguistic changes are really prominent. You have what has been described clinically as ‘empty speech’ where the person is dancing around the topic or not really providing a lot of content. We look at the proportion of nouns or content words, versus filler words or pronouns in Alzheimer's, while in other diseases such as depression, it might be more about tone of voice, pace of speech. We calculate almost 800 different metrics from every speech sample, which gives us insight into things like the pace of speech, the sounds of the voice, the words being used, how much the individual is repeating themselves and how much time they spend pausing, looking for words and so on.
How does speech differ between conditions such as Alzheimer’s, depression and schizophrenia?
There are some common features in neurocognitive diseases, such as speaking slower and pausing more. But then there are certain features that we think are more specific. There's also quite a bit of research on speech patterns in schizophrenia, for example, where speech is more disorganized and incoherent. In depression, a lot of the changes that have been reported in the literature and our research have to do with tone and pitch of voice and using negative words as well as the use of first-person pronouns. These patterns would allow us to differentiate between diseases.
How are you applying the findings and your technology to clinical studies and clinical settings?
The speech assessments are very short, usually less than five minutes, and can be done using a smartphone or a tablet. This is quite different from other traditional neuropsychological assessments that require a clinician to sit with someone in clinic for hours at a time and go through long tests. This allows for more flexibility in study design or trial design and allows investigators to collect data more frequently and even remotely with someone using a device at home. Adding a speech assessment or even just recording the assessments that are already being done means there’s no additional burden to the participant. We have worked in other, more hybrid studies where participants might do longer in-clinic testing days and in between those assessments use remote tools, including speech assessment. The hope is we can get much richer data, rather than simply relying on three or four clinic visits a year, which would give better insight into how that person is doing over a longer period of time.
We have been included as an exploratory endpoint in a number of trials for neurodegenerative disease, including Alzheimer's and frontotemporal dementia (FTD). These are largely retrospective research projects where our partners may have already collected speech data and they want to use our data for validation and exploration to see how speech analytics maps on to other clinical measures or other clinical phenotypes. We’re also partnering with research centers, hospitals and academic collaborators to prospectively collect these speech datasets to validate these tools for clinical trials.
Are there goals to use these tools in clinical trials to assess the impact of treatment?
We know there are limitations with the traditional endpoints used in these trials and the hope is that if speech is more sensitive, or if we collected at higher frequency, we may be able to pick up on changes with more sensitivity or sooner. In Alzheimer's trials where there have been mixed results where one endpoint might have a significant effect another doesn't, having additional measures might help bolster the evidence if there are signs of change.
There’s a huge appetite for innovation in endpoints and a lot of interest in speech among patients, caregivers and clinicians. Some of our partners are really innovative and excited to include new tools as exploratory endpoints and use these trials to learn and help develop the technology, and others have taken a more conservative approach. But the interest is there. It’s really about gathering more data, having more validation and getting more regulatory approvals to move this forward.
Where do you envision taking this technology in future?
While we’re still validating the technology and learning more, there is a lot of opportunity in early drug development, so go/no go decisions in early trials and being that extra exploratory endpoint. We hope to move forward with regulatory approvals, more consensus across the field and more big data sets so that we can get to a point where we are a primary or secondary endpoint in later stage trials. Eventually, we would like to get this into healthcare settings. If patients had a speech assessment as part of an annual checkup, that would be incredible longitudinal data and could potentially make the early detection tools a lot more accessible, especially if it’s just an app on a smartphone that people can speak into and get an alert of something potentially trending in the wrong direction. That could be really transformative for getting these solutions out to more people in a more accessible way.