The artificial intelligence program AlphaFold 3, rolled out by Google DeepMind and Isomorphic Labs earlier this month, has been called “groundbreaking,” holding transformative potential in drug discovery.
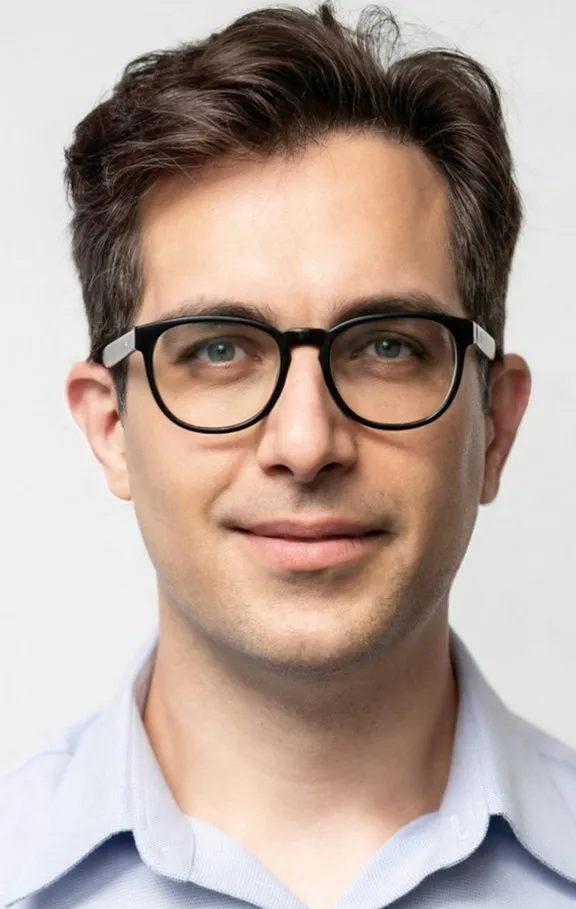
The hype is warranted, said Patrick Bangert, senior vice president of data, analytics, and AI at Searce, a cloud services company.
“It's a Nobel Prize-worthy invention,” he said.
AlphaFold 3’s capabilities, outlined in the journal Nature, build on two of its earlier AI models, enabling drug developers to quickly identify promising targets, potentially slashing the time to market by years.
Earlier versions of AlphaFold spurred a leap forward in drug development by rapidly and accurately predicting 3-D protein structures, a decades-long problem that had vexed computational biologists seeking to understand how shape affects protein function as a disease indicator. Earlier versions of AlphaFold, available through open-source code, were limited primarily by an inability to predict how proteins would interact with other molecules throughout the cell. Even so, they spurred significant progress.
“So far, millions of researchers globally have used AlphaFold 2 to make discoveries in areas including malaria vaccines, cancer treatments, and enzyme design,” according to the Google AlphaFold 3 announcement. “AlphaFold has been cited more than 20,000 times and its scientific impact recognized through many prizes, most recently the Breakthrough Prize in Life Sciences.”
The AlphaFold 3 program powers the AlphaFold server, which offers a web interface that allows non-coders to input the name of a protein or a nucleic acid and generate structure predictions, including critical information about joint structures with elements such as RNA, DNA, ligands and others.
“For the interactions of proteins with other molecule types we see at least a 50% improvement compared with existing prediction methods, and for some important categories of interaction we have doubled prediction accuracy,” according to Google. Still, accuracy for other applications, such as protein-RNA interactions, may be further off the mark.
Traditionally, scientists learned about protein structure using cost and time-intensive chemical laboratory trials, Bangert said. However, AlphaFold and competing programs, such as RoseTTaFold, now make the process quicker and easier.
“Instead of trialing a hundred options in the lab, physically, I can trial tens of thousands of options in silico,” he said. “While it still costs money, it's a small fraction and, of course, it's almost instantaneous.”
If a company typically takes ten years to get a drug across the finish line, this technology will allow them to do it in seven, he said.
It’s hard to find the downside of AlphaFold 3, Bangert said.
“This is actually one of those rare examples where I genuinely can't think of a drawback, mainly because this protein folding problem is so early in the process of drug development,” he said. “This is prior to doing any chemical experimentation, certainly prior to any kind of clinical trials, that even if you did make a mistake, it wouldn't matter because you wouldn't be impacting anything. So, there is only upside.”
That’s not to say companies using AlphaFold in drug development won’t face challenges.
“You have to have funding to be able to do this. And the second thing you need is data,” he said. “It's a protein fitting problem. You have the disease protein, and then you have the candidate protein and you're trying to fit them, which means that you have to have enough data of the disease protein available to you to be able to do that.”
That requires access to patient data, which brings regulatory hurdles, creates sizable paperwork headaches and makes health system partnerships necessary, Bangert said.
But while researchers have had difficulties finding qualified workers to carry out earlier-stage research, the technology may reduce that need. Rather than posing a threat to existing jobs, the AI program could become a pressure release valve for an area often stretched too thin, Bangert said.
Given its potential in life science research, DeepMind and Isomorphic’s CEO said this month the platform could eventually be a “multi-hundred billion” dollar business. And while AlphaFold 3 could identify promising compounds faster, the usual hurdles to market will remain.
“This isn't necessarily a Holy Grail to instantly make the drug development process more successful,” Bangert said. “You're still going to have the traditional challenges, but this is a piece of that puzzle that's fitting better now.”